Heart attack diagnosis may be improved by artificial intelligence
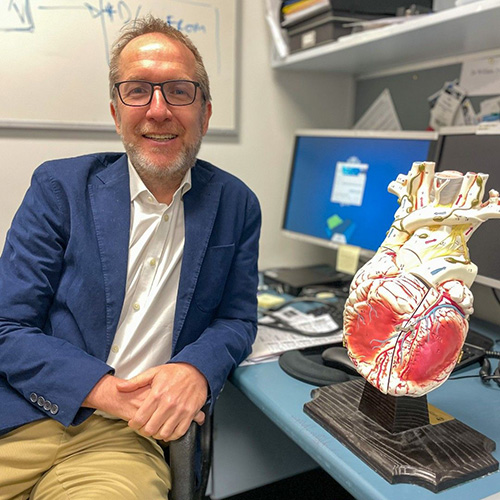
Image courtesy of the Royal Brisbane and Women’s Hospital
Researchers have developed a computer algorithm using artificial intelligence to diagnose heart attacks with better speed and accuracy than ever before. The new test could soon be used by doctors and greatly reduce hospital admissions to overcrowded Emergency Departments.
With an accuracy of 99.6%, the diagnostic tool known as CoDE-ACS was successfuly able to rule out a heart attack in twice as many patients as standard testing methods.
Prof. Nicholas Mills, British Heart Foundation Professor of Cardiology at the University of Edinburgh, is currently leading clinical trials in Scotland. AusHSI Clinical Director and RBWH cardiologist Prof. Will Parsonage, along with colleagues Prof. Louise Cullen and A/Prof. Jaimi Greenslade, are part of the international team which has tested the algorithm on 10,286 patients in six countries around the world.
According to Prof. Parsonage, while many patients come into ED with chest pain and symptoms of a heart attack, only 10-20% of those are actually having a heart attack. The new test will help identify which patients are safe to go home, and which need to stay in hospital for further tests.
The standard process for diagnosing a heart attack involves measuring levels of the protein troponin in the blood, with the same threshold used for every patient. However, this process does not account for factors that affect troponin levels, such as age, sex or pre-existing health conditions. This can affect the accuracy of heart attack diagnoses.
“The AI will learn from individual factors such as age, gender and blood test levels to help clinical staff in their decision making moving forward.”, said Prof. Parsonage.
CoDE-ACS has the potential to make emergency care more efficient and effective, and reduce misdiagnosis and inequalities across the population.
Research published on 11 May 2023 in the journal Nature Medecine.
Media contact
Sian Conway Lamb, 07 3138 6087, sian.conwaylamb@qut.edu.au